Two Professors of Mathematics Collaborated on a Publication Featured in the Journal Of Computational Neuroscience
POSTED ON: August 30, 2023
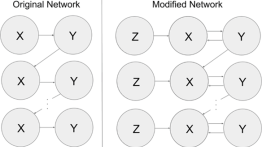
Figure 3 from the publication: A high-efficiency model indicating the role of inhibition in the resilience of neuronal networks to damage resulting from traumatic injury
Professor of Mathematics Stanislav Mintchev and Adjunct Professor Brian L. Frost-Laplante have collaborated on a publication in the Journal of Computational Neuroscience. The paper delves into the topic of "A high-efficiency model indicating the role of inhibition in the resilience of neuronal networks to damage resulting from traumatic injury."
The Journal of Computational Neuroscience is open to a wide range of submissions, including full-length original papers, rapid communications, and review articles discussing both theoretical and experimental research related to computations in the brain and nervous system. The journal particularly encourages papers that incorporate both theoretical and experimental approaches.
For additional information regarding this publication, please click here.
ABSTRACT
Recent investigations of traumatic brain injuries have shown that these injuries can result in conformational changes at the level of individual neurons in the cerebral cortex. Focal axonal swelling is one consequence of such injuries and leads to a variable width along the cell axon. Simulations of the electrical properties of axons impacted in such a way show that this damage may have a nonlinear deleterious effect on spike-encoded signal transmission. The computational cost of these simulations complicates the investigation of the effects of such damage at a network level. We have developed an efficient algorithm that faithfully reproduces the spike train filtering properties seen in physical simulations. We use this algorithm to explore the impact of focal axonal swelling on small networks of integrate and fire neurons. We explore also the effects of architecture modifications to networks impacted in this manner. In all tested networks, our results indicate that the addition of presynaptic inhibitory neurons either increases or leaves unchanged the fidelity, in terms of bandwidth, of the network’s processing properties with respect to this damage.