Three Cooper Alumni and Prof. Sam Keene published in the 2022 Biomedical Sensing and Analysis: Signal Processing in Medicine and Biology
POSTED ON: October 10, 2022
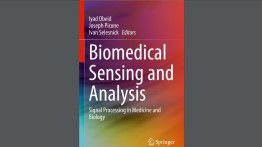
Image of the Biomedical Sensing and Analysis: Signal Processing in Medicine and Biology (2022) cover.
A 2020 senior project by three electrical engineering alumni Jonathan Pedoeem EE’20, Guy Bar Yosef EE’20, Shifra Abittan EE’20, and Professor of Electrical Engineering Sam Keene was published as a chapter on “TABS: Transformer Based Seizure Detection” in the Biomedical Sensing and Analysis: Signal Processing in Medicine and Biology (2022).
Read more about the research here.
ABSTRACT
In this chapter we explain our development of a novel deep learning model that can predict seizures from an EEG signal. We cover our approach and data pipeline, as well as the iterations our model took before settling into its final form. As part of this project, we participated in the Neureka 2020 challenge, a month-long challenge to detect seizures from Temple University’s EEG corpus, the TUH EEG seizure dataset. Our model is a neural network that includes fully connected layers, convolutional layers, and most notably a transformer layer. As such we call it TABS, after Transformer Based Seizure Detection.
Instead of aiming to achieve the maximum sensitivity, the Neureka challenge placed particular emphasis on limiting the number of false positives coming out of models, or reducing the places in which background signal is erroneously labeled as a seizure. As a result, our model is intended to act as a tool to aid neurologists as opposed to supplanting them completely. The goal is to allow a doctor to move more quickly through EEG data, in order to treat more patients and devote more time towards treatment. In the Neureka challenge TABS scored a sensitivity of 9.03% and a false alarm rate of 31.21 per 24 h when evaluated using the Time-Aligned Event Scoring metric.