Prof. Topper Co-Authors Publication on Machine Learning in Chemistry
POSTED ON: September 25, 2017
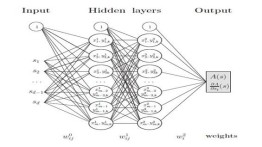
Last year while on sabbatical, Professor Robert Topper approached NYU Professor Mark Tuckerman, an internationally recognized expert in chemical physics, and proposed that he assist his group with their work. They struck up a collaboration based on the work of Dr. Elia Schneider, a postdoctoral researcher in Tuckerman's group who was developing the use of artificial neural networks to "learn" the immense amount of data produced by molecular dynamics simulations. This research also had important contributions from visiting high school student Luke Dai and postdoctoral researcher Christof Drechsel-Grau. The work was recently accepted for publication in Physical Review Letters, one of the most widely disseminated scientific journals in the world, and will be published sometime in Fall 2017.
Professor Topper is currently working with Schneider, Tuckerman, and members of the Tuckerman group on further research in this field. This project's success shows that machine learning methods can be a valuable tool in the prediction and analysis of the thermodynamic properties of compounds of biological importance as well as solid-solid phase transitions.
Title: "Stochastic Neural Network Approach for Learning High-Dimensional Free Energy Surfaces"
Abstract:
The generation of free energy landscapes corresponding to conformational equilibria in complex molecular systems remains a significant computational challenge. Adding to this challenge is the need to represent, store, and manipulate the often high-dimensional surfaces that result from rare-event sampling approaches employed to compute them. In this Letter, we propose the use of artificial neural networks as a solution to these issues. Using specific examples, we discuss network training using enhanced-sampling methods and the use of the networks in the calculation of ensemble averages.